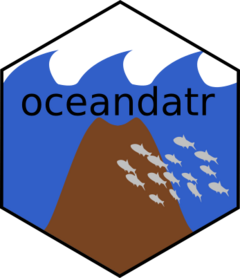
Get a set of feature data for a spatial grid
get_features.Rd
This is a wrapper of get_bathymetry()
, get_seamounts_buffered()
, get_knolls()
, get_geomorphology()
, get_coral_habitat()
, and get_enviro_regions()
. See the individual functions for details.
Usage
get_features(
spatial_grid = NULL,
raw = FALSE,
features = c("bathymetry", "seamounts", "knolls", "geomorphology", "corals",
"enviro_regions"),
bathy_resolution = 1,
seamount_buffer = 30000,
antipatharia_threshold = 22,
octocoral_threshold = 2,
enviro_clusters = NULL,
max_enviro_clusters = 6,
antimeridian = NULL
)
Arguments
- spatial_grid
sf
orterra::rast()
grid, e.g. created usingget_grid()
. Alternatively, if raw data is required, ansf
polygon can be provided, e.g. created usingget_boundary()
, and setraw = TRUE
.- raw
logical
if TRUE,spatial_grid
should be ansf
polygon, and the raw feature data in that polygon(s) will be returned. Note that this will be a list object, since raster andsf
data may be returned.- features
a vector of feature names, can include: "bathymetry", "seamounts", "knolls", "geomorphology", "corals", "enviro_regions"
- bathy_resolution
numeric
; the resolution (in minutes) of data to pull from the ETOPO 2022 Global Relief model. Values less than 1 can only be 0.5 (30 arc seconds) and 0.25 (15 arc seconds)- seamount_buffer
numeric
; the distance from the seamount peak to include in the output. Distance should be in the same units as the area_polygon or spatial_grid provided, use e.g.sf::st_crs(spatial_grid, parameters = TRUE)$units_gdal
to check what units your planning grid or area polygon is in (works for raster as well as sf objects)- antipatharia_threshold
numeric
between 0 and 100; the threshold value for habitat suitability for antipatharia corals to be considered present (default is 22, as defined in Yesson et al., 2017)- octocoral_threshold
numeric
between 0 and 7; the threshold value for how many species (of 7) should be predicted present in an area for octocorals to be considered present (default is 2)- enviro_clusters
numeric
; the number of environmental regions to cluster the data into - to be used when a clustering algorithm is not necessary (default is NULL)- max_enviro_clusters
numeric
; the maximum number of environmental regions to try when using the clustering algorithm (default is 8)- antimeridian
Does
spatial_grid
span the antimeridian? If so, this should be set toTRUE
, otherwise set toFALSE
. If set toNULL
(default) the function will try to check if data spans the antimeridian and set this appropriately.
Value
If raw = TRUE
, a list of feature data is returned (mixed raster and sf
objects). If a spatial_grid
is supplied, a multi-layer raster or sf
object of gridded data is returned, depending on the spatial_grid
format.
Examples
# Grab EEZ data first
bermuda_eez <- get_boundary(name = "Bermuda")
#> Cache is fresh. Reading: /tmp/Rtmpj5K8Rh/eez-2205f12f/eez.shp
#> (Last Modified: 2024-09-11 02:15:56.249906)
# Get raw data for Bermuda's EEZ
raw_data <- get_features(spatial_grid = bermuda_eez, raw = TRUE)
#> Getting depth zones...
#> This may take seconds to minutes, depending on grid size
#> Getting seamount data...
#> Spherical geometry (s2) switched off
#> although coordinates are longitude/latitude, st_intersection assumes that they
#> are planar
#> Warning: attribute variables are assumed to be spatially constant throughout all geometries
#> Warning: st_buffer does not correctly buffer longitude/latitude data
#> dist is assumed to be in decimal degrees (arc_degrees).
#> Spherical geometry (s2) switched on
#> Getting knoll data...
#> Spherical geometry (s2) switched off
#> although coordinates are longitude/latitude, st_intersection assumes that they
#> are planar
#> Warning: attribute variables are assumed to be spatially constant throughout all geometries
#> Spherical geometry (s2) switched on
#> Getting geomorphology data...
#> Getting coral data...
#> Getting environmental regions data... This could take several minutes
# Get feature data in a spatial grid
bermuda_grid <- get_grid(boundary = bermuda_eez, crs = '+proj=laea +lon_0=-64.8108333 +lat_0=32.3571917 +datum=WGS84 +units=m +no_defs', resolution = 20000)
features_gridded <- get_features(spatial_grid = bermuda_grid)
#> Getting depth zones...
#> This may take seconds to minutes, depending on grid size
#> Getting seamount data...
#> Spherical geometry (s2) switched off
#> although coordinates are longitude/latitude, st_intersection assumes that they
#> are planar
#> Warning: attribute variables are assumed to be spatially constant throughout all geometries
#> Spherical geometry (s2) switched on
#> Getting knoll data...
#> Spherical geometry (s2) switched off
#> although coordinates are longitude/latitude, st_intersection assumes that they
#> are planar
#> Warning: attribute variables are assumed to be spatially constant throughout all geometries
#> Spherical geometry (s2) switched on
#> Getting geomorphology data...
#> Getting coral data...
#> Getting environmental regions data... This could take several minutes
terra::plot(features_gridded)